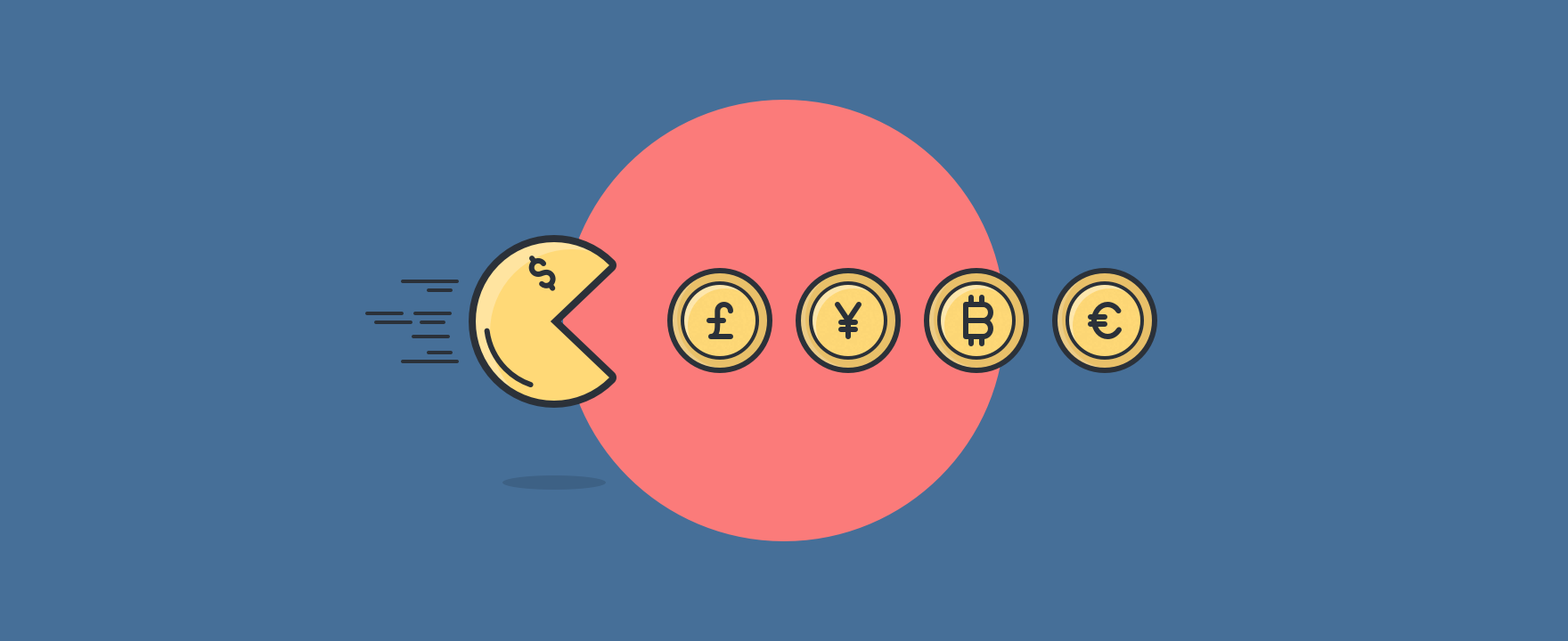
Whether you have come up with a brilliant new idea for a game, completed a prototype, or are halfway through the intended post-launch life cycle, the hardest part is to convert creative success into a financial one. A high-quality game may not always serve as the basis for attracting more investment to your company. The product must justify its development costs and generate a solid income. Here investors often run into a problem: neither game studios nor the largest investment banks may be able to accurately predict a game company’s cash flow in a timely manner, which in some eyes renders the entire sector quite risky. One of the reasons for this are inaccurate or even wishful sale forecasts. To reduce volatility and better understand an individual game’s potential, it is very important to make the most accurate project assessment at the earliest possible stage of its development.
Game potential valuation is carried out both independently and by specialized companies with relevant experience. There is a lot of information available online on how to perform a quick sales forecast for a future project. The overall challenge is to gather the right data and make good use of one’s analytical skills.
GID-R — a method for evaluating future game sales
The Game-In-Development-Report (GID-R) sales potential assessment methodology developed by the Data40 team allows to assess the sales potential of games at various stages of development. The key advantage of GID-R is the combination of academic (quantitative) methods and expert opinion, which enables you to evaluate the forecast from different angles. The method is scalable, expandable, and permits the introduction of new evaluation models and hypothesis testing.
An important step in GID-R evaluation is gathering the lists of known competitors and game references for your project. Information about competitors and references is valuable as it provides more systematic and representative market data than consumer research.
When collecting references, it is recommended to use several methods:
- Search through the “Similar Games” section of your project’s Steam page;
- Add references you already know about;
- Check with SteamPeek.hu;
- Include other platforms that offer similar games.
As an example, we used the data collected for Stray, received a month before the game’s eventual release on Steam.
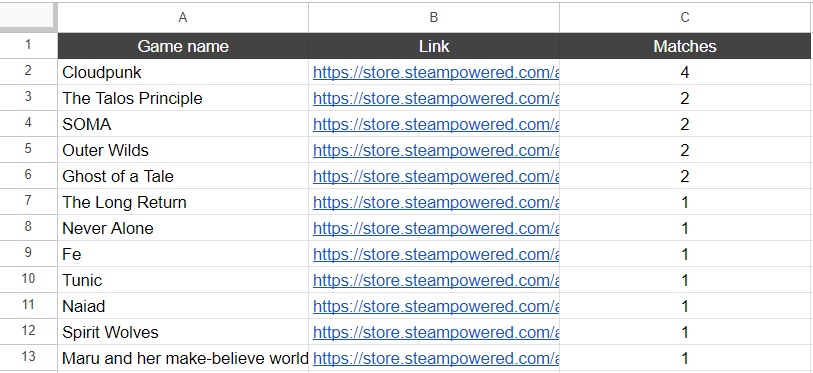
Evaluating the market through the lens of supply rather than demand allows one to account for more parameters and ultimately improves forecast quality. An assessment of competitors’ current performance will enable you to predict the sales volume for your own game in the same segment. With the GID-R method, the assessment of revealed competitors’ audience can be carried out according to several models.
Model 1: Steam Subscribers
- Gather Steam group subscribers for all references in question;
- Find out the number of copies sold on Steam via references. We typically use the SteamSpy service for this;
- Compare the Steam group subscriber data and the number of copies sold for reference games. Find the average number of Steam group subscribers and the average number of sales for all references;
- Calculate the sales potential of your own game using the following formula:
- Steam subscribers for your game / Average subscribers for collected references * Average owners for collected references.
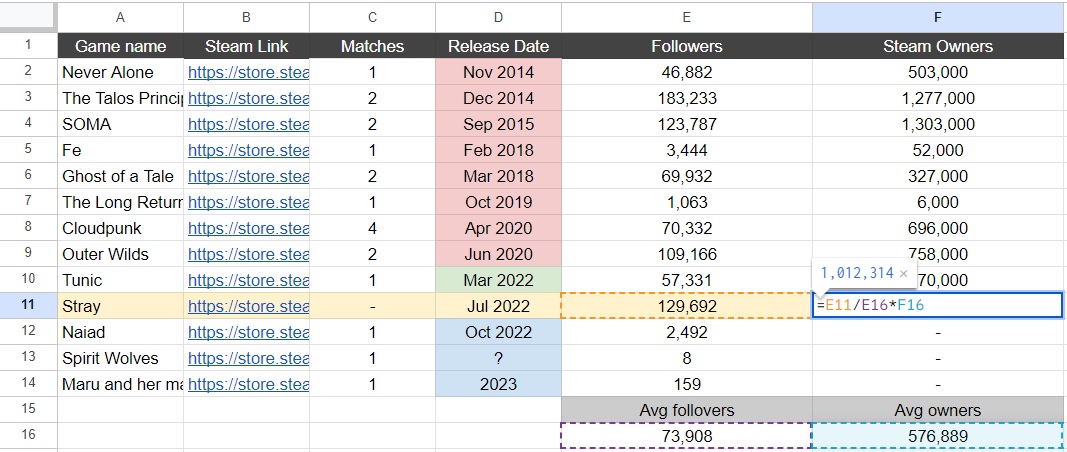
Model 2: YouTube Views
- Check the view numbers for the TOP-10 YouTube videos per collected reference/competitor;
- Compare data on the numbers of YouTube views and copies sold for referenced games. Find the average number of sales per 1 million views for each of the references with the following formula:
- Number of Steam sales for collected reference / Number of YouTube views for the TOP-10 videos per individual reference, rounded to the nearest million.
- Find the average number of sales per 1 million views for all considered reference games;
- Calculate the sales potential of your own game using this formula:
- Number of YouTube views for the TOP 10 videos of your game rounded to the nearest million * Average number of sales per 1 million views for all considered reference games.
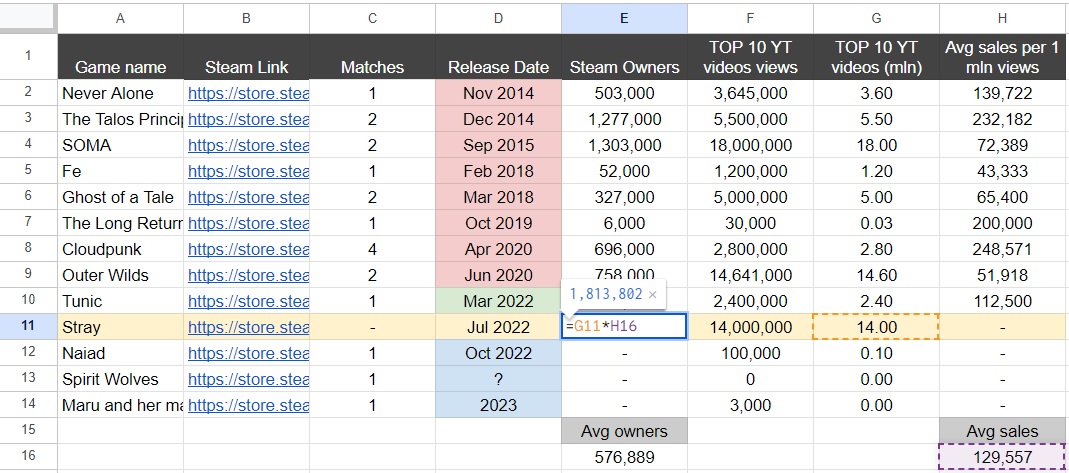
Model 3: Website Traffic
- The model is only relevant when forecasting prior to a project’s official release and can be excluded if the game has already gone live;
- The model is usually built upon a truncated sample, which makes it less reliable when compared to the models described above;
- Collect the monthly traffic history for reference games at the time of their release. We recommend using the following services:
- Compare pre-release traffic data (including several slices at once) with the number of copies sold for reference games. Find the average amount of traffic before and at the time of release as well as the average sales numbers for all references;
- Determine the traffic for your own game’s website before the upcoming release;
- Calculate the sales potential of your game with this formula:
- Your game’s website traffic before upcoming release / Average pre-release traffic for collected references * Average reference game owners.
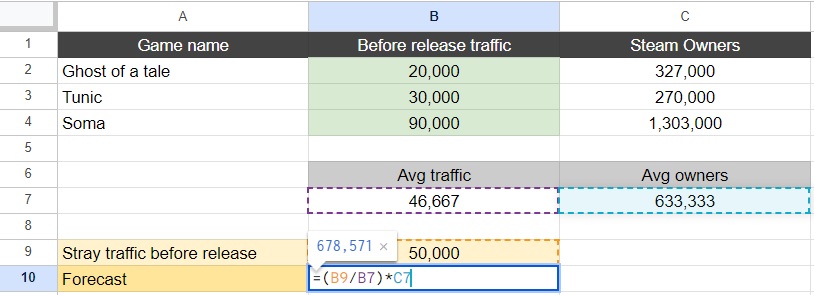
Calculating final Steam sales prediction based on collected data
Predictions based on all models should be compared to determine the current final Steam sales forecast.
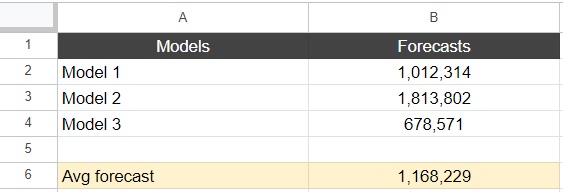
By the time Stray was released, a month after these calculations were made, its metrics and performance were rapidly increasing. The applied models have successfully predicted future growth in the game’s sales, which is now confirmed by the number of Steam users who own a copy of Stray.
Updating Stray’s data within these pre-prepared models at the time of the game’s release would allow us to further predict future sales figures that would differ from those we anticipated a month before the project became available to the general audience.
Metrics will inevitably change in the process of improving the game and due to its marketing activities, but all that you need to do is adjust corresponding values inside the assembled model table in order to track future sales dynamics.
Improving game sales forecasting models
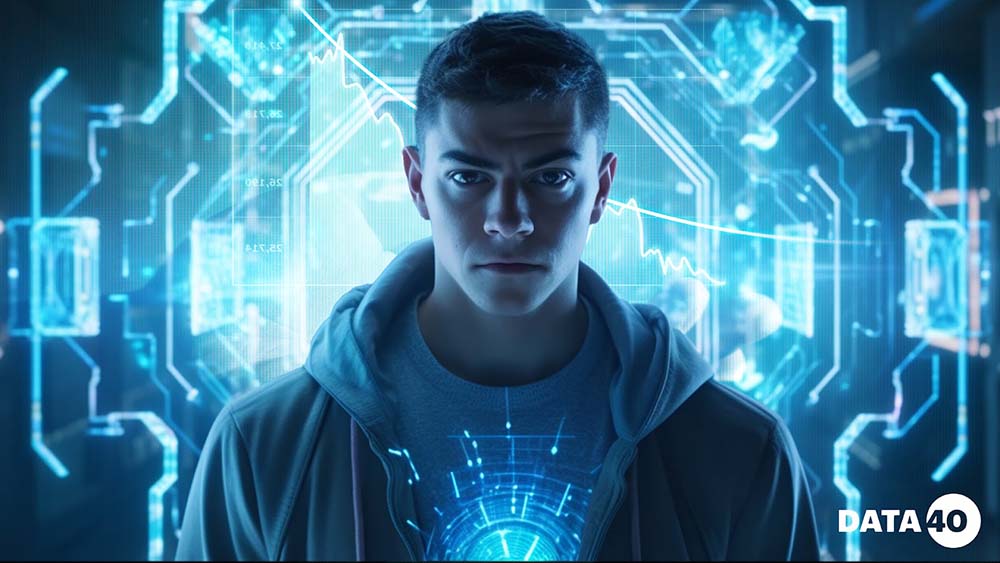
The GID-R method is flexible and can be extended with additional parameters both within an existing model or by introducing a new one to increase the reliability of generated forecasts.
Additionally, the method allows building forecasts for more than just the game’s current performance. In our models (for example with the TOP-10 viewed YouTube videos), we can augment the game’s performance, enabling us to make an assumption of future sales and compare the results against third-party estimates, such as sales forecasts provided by publisher experts.
The Data40 team is constantly upgrading our GID-R methodology by developing and expanding several distinct models. We are already offering sales forecasts not only for Steam but all other available platforms. Unfortunately, it is impossible to describe all the assembled models and their parameters in one short article. However, the approach described here should enable any developer to independently predict their game’s sales potential.